UC Santa Cruz researchers build AI to prevent drownings
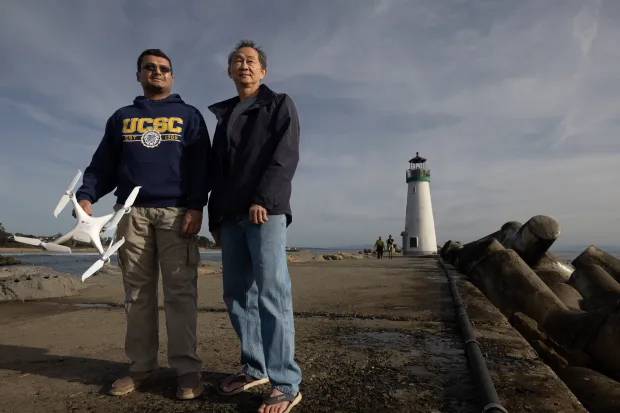
As the winter swell approaches, researchers at UC Santa Cruz are working on potentially life-saving artificial intelligence (AI) technology. In collaboration with the National Oceanic and Atmospheric Administration (NOAA) and funded by UC Santa Cruz’s Center for Coastal Climate Resilience, Alex Pang and his team are developing algorithms, which are sets of programmed instructions. These algorithms aim to monitor changes in the shoreline, identify rip currents, and alert lifeguards to potential hazards, with the goal of enhancing beach safety and saving lives.
The idea for this project originated while Pang was windsurfing with friends. They would point out rip currents that were challenging for him to discern visually. Recognizing the difficulty in detecting rip currents with the untrained eye, Pang envisioned the utility of computer technology for this purpose. He initiated the rip current detection project in 2015, a year before UC Santa Cruz experienced the tragic loss of two students to drowning.
Pang highlighted the added urgency to develop this capability after the drowning incidents, emphasizing the potential impact on beach safety. NOAA defines rip currents as “powerful, narrow channels of fast-moving water” that pose a risk by pulling swimmers into deep water, leading to fatigue and the possibility of drowning as individuals attempt to fight against the current. Santa Cruz Marine Safety has reported 10 drowning deaths in the past decade, including two in 2023.
The National Weather Service categorizes rip currents as the third most perilous among all weather hazards, trailing behind heat and flooding.
Detecting rip currents from the shore can be challenging, and they can manifest unexpectedly. Pang’s team considered various approaches for rip detection and ultimately opted for a machine-learning-based system, akin to the obstacle detection systems employed in self-driving cars.
Machine learning, a form of artificial intelligence, refers to a machine’s capacity to make decisions based on the information it has been provided. Scientists trained their rip current detector by presenting it with a set of images containing rip currents and others without, allowing the system to learn the common attributes of a rip current. Following this training, the detector can identify rip currents in live video streams.
The team led by Pang collaborated with NOAA to develop and refine their detection methods. They installed a streaming webcam at Walton Lighthouse, offering views of Seabright and Twin Lakes beaches, with the support of the Santa Cruz Harbor Office, O’Neill Sea Odyssey, and the U.S. Coast Guard. Currently, the machine learning model is utilized to process and detect rip currents in real-time through the live video feed of these beaches.

Pang’s rip current detection model will generate a dataset of rip current observations, contributing to the validation and enhancement of NOAA’s existing forecast model developed by Greg Dusek, a physical oceanographer at NOAA. Dusek explained that the forecast model evaluates the risk of rip current development based on factors such as wave height, wave direction, tide, and the presence of sand bars, providing a likelihood prediction similar to other weather forecasts.
Once the rip current detector model proves reliable with minimal false alarms, the research team aims to create an alert system customizable for lifeguards based on their specific needs. Ideally, this system will send alerts to lifeguards only when individuals are detected in rip currents and can distinguish between people and surfers if necessary.